Abstract
As artificial intelligence (AI) evolves, the Unified Agent emerges as a transformative concept that integrates hybrid supervision and Crew AI methodologies into a single, cohesive framework. By blending human oversight, modular subroutines, and self-supervisory mechanisms, the Unified Agent aims to enhance transparency, scalability, and ethical alignment in AI systems. This paper explores the design, functionality, and applications of the Unified Agent, highlighting its potential to redefine the landscape of autonomous systems through a collaborative approach to complexity.
1. Introduction
Artificial intelligence has reached a crossroads where the demands for transparency, accountability, and ethical alignment often clash with the need for efficiency and scalability. Traditional models, such as Crew AI—a system of multiple collaborative agents—and hybrid supervision—a blend of human and machine oversight—offer partial solutions to these challenges. The Unified Agent concept seeks to integrate the strengths of both approaches, creating a single, sophisticated system that embodies the collaborative dynamics of Crew AI while benefiting from the control and transparency of hybrid supervision.
2. The Foundations of the Unified Agent
2.1 Hybrid Supervision
Hybrid supervision involves a collaborative framework where human intervention and independent AI modules ensure accuracy, ethical compliance, and operational transparency. Key components include:
-
Human Oversight: Provides contextual and ethical judgment.
-
Independent AI Modules: Validate and refine primary outputs.
-
Access Panels: Facilitate user interaction and transparency in decision-making processes.
2.2 Crew AI Methodologies
Crew AI systems comprise multiple specialized agents working in concert to tackle complex tasks. Each agent brings unique capabilities, creating a system that is:
-
Specialized: Tailored for distinct functions.
-
Collaborative: Designed for inter-agent synergy.
-
Resilient: Able to handle system-wide redundancies.
3. Defining the Unified Agent
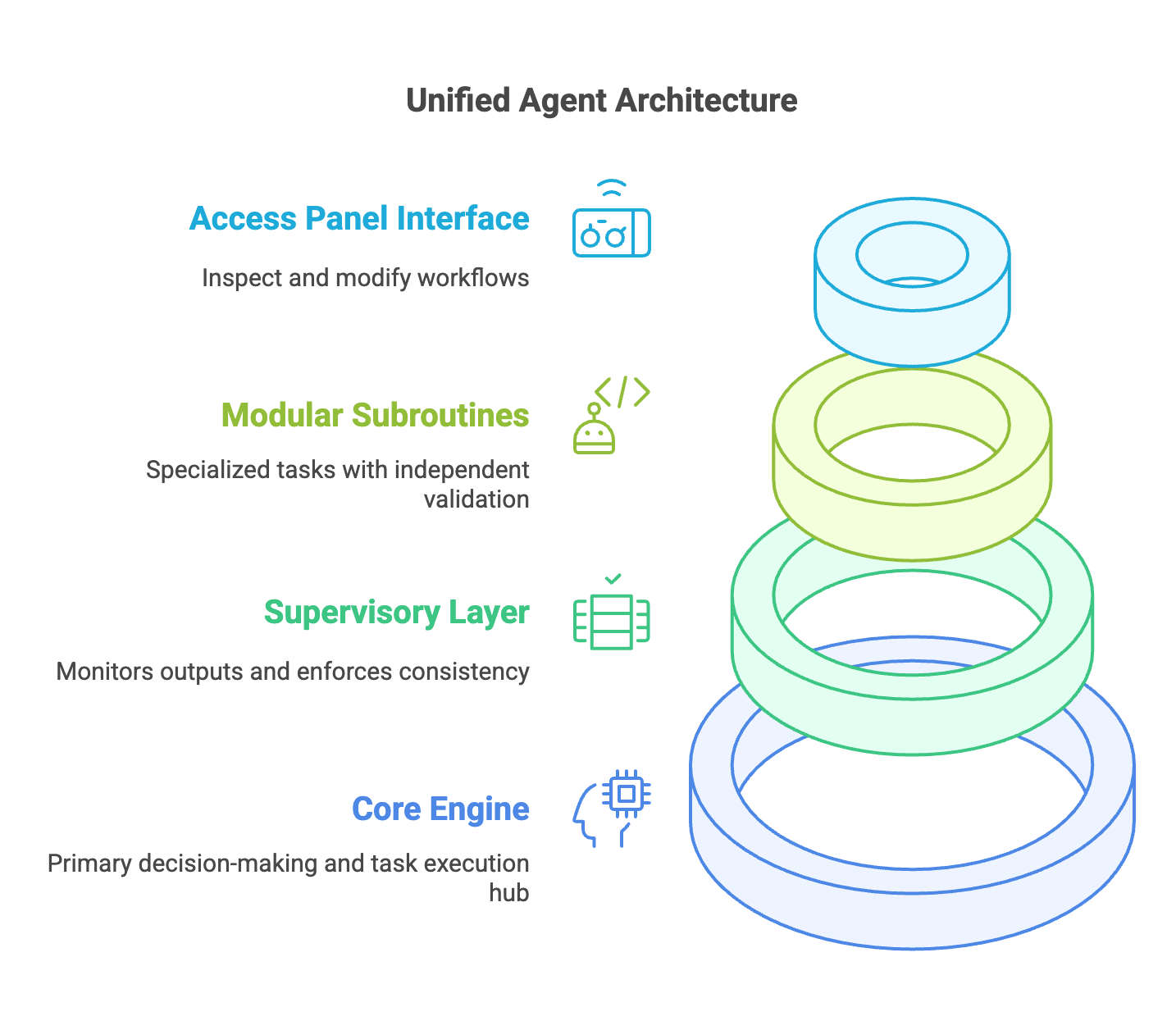
The Unified Agent combines these methodologies within a single AI framework, leveraging internal modularity and reflexive feedback mechanisms to simulate the dynamics of a multi-agent system.
3.1 Core Principles
- Modularity: Integrates specialized subroutines for tasks such as data validation, ethical evaluation, and iterative optimization.
- Self-Supervision: Employs internal feedback loops to monitor and refine its processes.
- Transparency: Utilizes Access Panels for real-time user interaction and oversight.
3.2 Architecture
The Unified Agent architecture consists of:
- Core Engine: Handles primary decision-making and task execution.
- Supervisory Layer: Monitors outputs and enforces consistency.
- Modular Subroutines: Perform specialized tasks with independent validation mechanisms.
- Access Panel Interface: Allows stakeholders to inspect and modify workflows.
4. Bridging Hybrid Supervision and Crew AI
4.1 Integrative Design
The Unified Agent’s design borrows from Crew AI by replicating its collaborative dynamics within a single agent, achieved through modularity. It also incorporates hybrid supervision by embedding human oversight and independent validation into its workflow.
4.2 Collaborative Dynamics
By simulating multiple agents through modular subroutines, the Unified Agent maintains the advantages of specialization and redundancy inherent in Crew AI. Reflexive feedback loops ensure continuous refinement, mirroring the iterative nature of hybrid supervision.
5. Functionalities of the Unified Agent
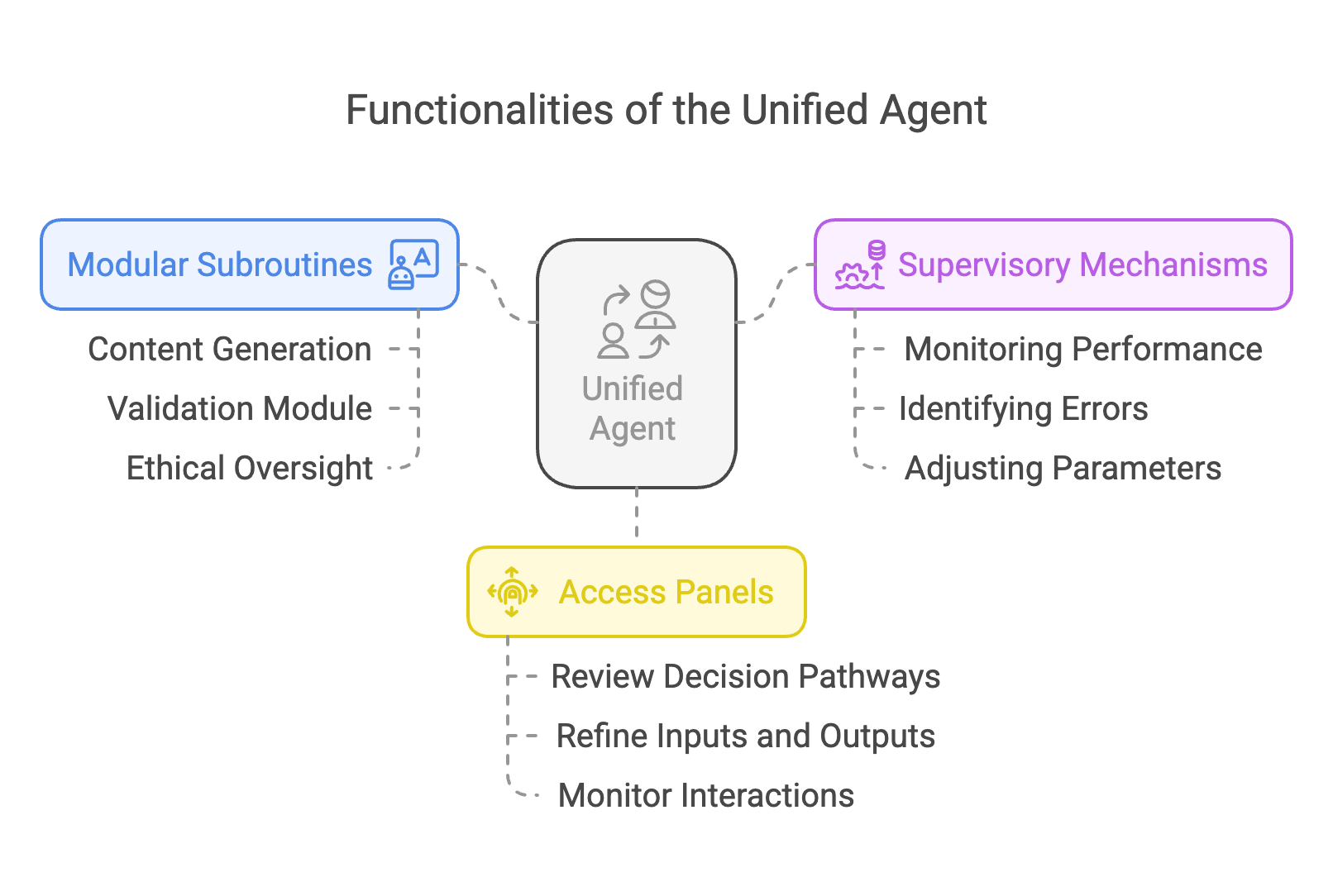
5.1 Modular Subroutines
Each subroutine operates as a semi-autonomous entity within the Unified Agent. Key modules include:
- Content Generation: Produces task-specific outputs.
- Validation Module: Ensures accuracy and alignment with predefined metrics.
- Ethical Oversight: Evaluates outputs against ethical guidelines.
5.2 Supervisory Mechanisms
Supervisory layers provide oversight by:
- Monitoring subroutine performance.
- Identifying inconsistencies or errors.
- Adjusting parameters based on real-time feedback.
5.3 Access Panels
Access Panels enhance transparency and control by allowing users to:
- Review decision-making pathways.
- Refine inputs and outputs.
- Monitor subroutine interactions.
6. Comparative Analysis
Feature | Hybrid Supervision | Crew AI | Unified Agent |
---|---|---|---|
Structure | Human-AI collaboration | Multiple AI agents | Single AI with modularity |
Transparency | High | Moderate | High |
Scalability | Moderate | Limited by coordination | High |
Error Mitigation | Human intervention | Redundancy | Reflexive feedback loops |
Collaboration | Between humans and AI | Between agents | Internal modular synergy |
7. Applications of the Unified Agent
7.1 Healthcare
In healthcare, the Unified Agent can streamline diagnostics by integrating modules for patient data analysis, symptom evaluation, and treatment planning. Access Panels enable clinicians to refine outputs, ensuring accuracy and ethical compliance.
7.2 Finance
For fraud detection, the Unified Agent combines pattern recognition, validation, and anomaly detection within a unified framework. Reflexive feedback and Access Panels allow financial analysts to identify and address false positives efficiently.
7.3 Education
In personalized learning environments, the Unified Agent tailors content delivery, monitors student progress, and provides feedback. Supervisory layers ensure that recommendations align with educational objectives.
8. Ethical Considerations
8.1 Accountability
The Unified Agent’s transparency mechanisms establish clear accountability pathways, ensuring stakeholders understand and trust the system’s decisions.
8.2 Bias Mitigation
Independent validation modules and Access Panels enable stakeholders to identify and rectify biases, fostering fair and equitable outcomes.
8.3 Governance
Robust governance frameworks are essential to maintain the Unified Agent’s alignment with societal values and ethical principles.
9. Implementation Framework
9.1 Design and Deployment
- Define Modular Tasks: Identify critical functions and design specialized subroutines.
- Integrate Supervisory Layers: Implement internal feedback mechanisms.
- Develop User Interfaces: Build Access Panels for transparency and control.
9.2 Training and Optimization
- Iterative Refinement: Use reflexive feedback loops for continuous improvement.
- Stakeholder Training: Educate users on interacting with the system.
- Regular Audits: Conduct periodic evaluations to ensure alignment and efficacy.
10. Conclusion
The Unified Agent represents a significant step forward in AI design, merging the strengths of hybrid supervision and Crew AI into a scalable, transparent, and ethical framework. By integrating modular subroutines, supervisory layers, and Access Panels, the Unified Agent offers a cohesive solution to the challenges of modern AI systems. As applications continue to expand, this approach holds the potential to redefine the boundaries of autonomy and collaboration in artificial intelligence.
References
- Leibo, J. Z., et al. (2017). “Multi-agent reinforcement learning in sequential social dilemmas.” Proceedings of the 16th Conference on Autonomous Agents and MultiAgent Systems.
- Dietterich, T. G. (2000). “Ensemble methods in machine learning.” International Workshop on Multiple Classifier Systems.
- Binns, R. (2018). “Fairness in machine learning: Lessons from political philosophy.” Proceedings of the 2018 Conference on Fairness, Accountability, and Transparency.
- Wachter, S., Mittelstadt, B., & Floridi, L. (2017). “Why a right to explanation of automated decision-making does not exist in the General Data Protection Regulation.” International Data Privacy Law.
- Doshi-Velez, F., & Kim, B. (2017). “Towards a rigorous science of interpretable machine learning.” arXiv preprint arXiv:1702.08608.